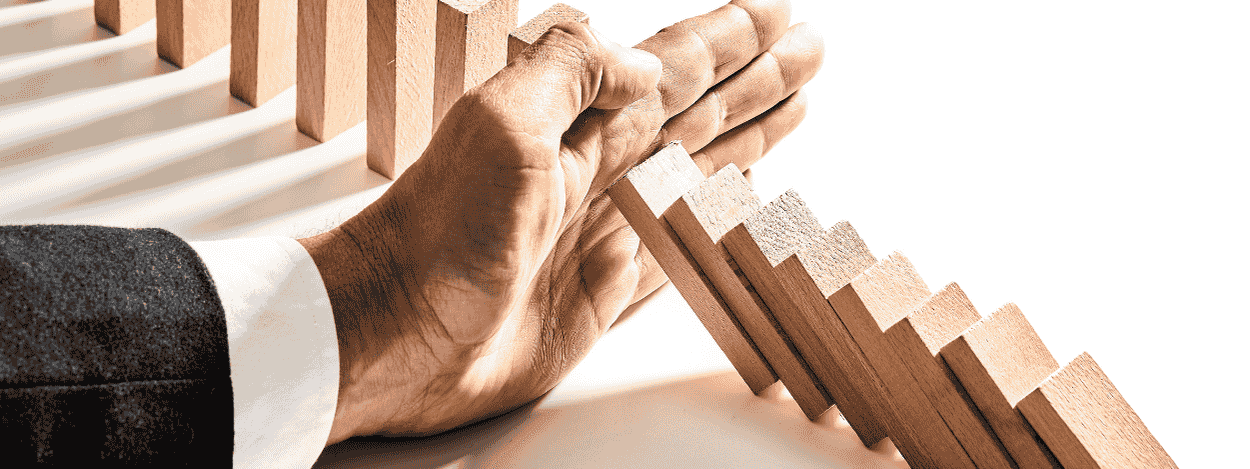
AI and Machine Learning in Enterprise Risk Management (ERM): Transforming Risk Assessment and Mitigation
In today’s rapidly evolving business landscape, organizations face a variety of risks that can impact their operations, reputation, and financial stability. Enterprise Risk Management (ERM) has become an essential strategic approach that helps businesses identify, assess, and mitigate potential risks. Traditionally, ERM relied on manual processes, historical data, and static risk models. However, the advent of Artificial Intelligence (AI) and Machine Learning (ML) has revolutionized the field, offering predictive insights, automation, and real-time risk monitoring. This article explores how AI and ML are transforming risk assessment and mitigation in ERM, highlighting key benefits, challenges, and future trends.
Understanding AI and Machine Learning in ERM
AI refers to the ability of machines to perform tasks that typically require human intelligence, such as problem-solving, pattern recognition, and decision-making. Machine Learning, a subset of AI, involves training algorithms to recognize patterns in data and make predictions or decisions based on those patterns.
In the context of ERM, AI and ML analyze vast amounts of structured and unstructured data to detect emerging risks, predict outcomes, and recommend risk mitigation strategies. These technologies enhance traditional risk management practices by providing proactive and data-driven insights that improve decision-making and resilience.
Courses by Aztech:
- Certificate in Enterprise Risk Management Best Practices
- The Complete Course in Enterprise Risk Management (ERM)
Key Applications of AI and ML in ERM
-
Automated Risk Identification
One of the most significant benefits of AI and ML in ERM is their ability to identify risks automatically. Traditional risk management methods rely on human judgment and past experiences, which can lead to biases and blind spots. AI-driven systems continuously scan data from multiple sources, including financial reports, market trends, social media, and regulatory updates, to detect potential risks before they materialize.
-
Predictive Risk Analytics
Machine learning models can analyze historical data and identify patterns that indicate potential risks. By leveraging predictive analytics, businesses can anticipate future risks and take proactive measures to mitigate them. For example, financial institutions use AI to predict credit defaults, while supply chain managers use ML to forecast disruptions due to geopolitical events or natural disasters.
-
Real-time Risk Monitoring and Alerts
AI-powered ERM systems provide real-time risk monitoring by continuously analyzing internal and external data sources. These systems generate alerts when anomalies or potential threats are detected, enabling organizations to respond swiftly. For instance, cybersecurity firms use AI to detect and prevent cyber threats by identifying unusual patterns in network traffic.
-
Fraud Detection and Prevention
Fraud is a significant concern for businesses, particularly in the financial sector. AI and ML enhance fraud detection by analyzing transaction patterns and flagging suspicious activities. By using real-time data analysis and behavioral analytics, AI can identify fraudulent transactions and reduce false positives, thereby improving efficiency in fraud prevention.
-
Regulatory Compliance and Risk Reporting
Regulatory compliance is a critical aspect of ERM, as organizations must adhere to various legal and industry standards. AI-driven compliance tools help businesses monitor regulatory changes, automate reporting, and ensure adherence to compliance requirements. Natural Language Processing (NLP) enables AI to analyze legal documents, identify relevant regulations, and provide compliance recommendations.
-
Enhanced Decision-making with AI-driven Insights
AI and ML enable data-driven decision-making by providing actionable insights into potential risks. These insights help risk managers evaluate the impact of different risk scenarios and choose the most effective mitigation strategies. Advanced visualization tools powered by AI make it easier for executives to interpret risk data and make informed decisions.
Benefits of AI and ML in ERM
-
Improved Accuracy and Efficiency
AI and ML reduce human errors in risk assessment by analyzing vast amounts of data with precision. Automated processes save time and resources, allowing risk management teams to focus on strategic planning rather than manual data analysis.
-
Proactive Risk Management
Unlike traditional risk management approaches that are often reactive, AI and ML enable organizations to predict and prevent risks before they escalate. This proactive approach enhances business resilience and minimizes potential losses.
-
Scalability and Adaptability
AI-driven ERM systems can scale with business growth and adapt to changing risk environments. Machine learning models continuously learn from new data, improving their accuracy and relevance over time.
-
Cost Savings
By automating risk assessment and mitigation, AI reduces operational costs associated with manual risk management processes. Businesses can allocate resources more efficiently and reduce financial losses due to unforeseen risks.
Challenges of Implementing AI and ML in ERM
-
Data Quality and Availability
AI and ML models require large amounts of high-quality data to function effectively. Inconsistent or incomplete data can lead to inaccurate risk assessments, making data management a critical challenge for organizations.
-
Complexity and Implementation Costs
Integrating AI into ERM systems requires significant investment in technology, infrastructure, and skilled personnel. Small and medium-sized enterprises (SMEs) may struggle with the costs and technical complexities of AI adoption.
-
Regulatory and Ethical Concerns
AI-driven risk management raises ethical and regulatory concerns, such as data privacy, algorithmic bias, and transparency. Organizations must ensure compliance with data protection laws and implement ethical AI practices to maintain trust.
-
Human Oversight and Interpretability
While AI enhances risk management, human oversight remains essential. AI models can be complex and difficult to interpret, making it crucial for risk managers to validate AI-driven insights and ensure alignment with business objectives.
Future Trends in AI and ML for ERM
-
Explainable AI (XAI) in Risk Management
To address the challenge of AI interpretability, Explainable AI (XAI) is gaining traction. XAI provides transparent and understandable AI models, enabling risk managers to trust and validate AI-generated insights.
-
AI-powered Risk Simulation and Scenario Analysis
Advanced AI models will increasingly be used to simulate various risk scenarios and assess their potential impact. These simulations help organizations develop robust risk mitigation strategies.
-
Integration of Blockchain with AI-driven ERM
Blockchain technology enhances transparency and security in risk management by providing immutable and tamper-proof records. Integrating AI with blockchain can further improve fraud detection and regulatory compliance.
-
AI-driven Cyber Risk Management
As cyber threats continue to evolve, AI will play a crucial role in enhancing cybersecurity risk management. AI-driven threat intelligence platforms will provide real-time threat detection and automated incident response